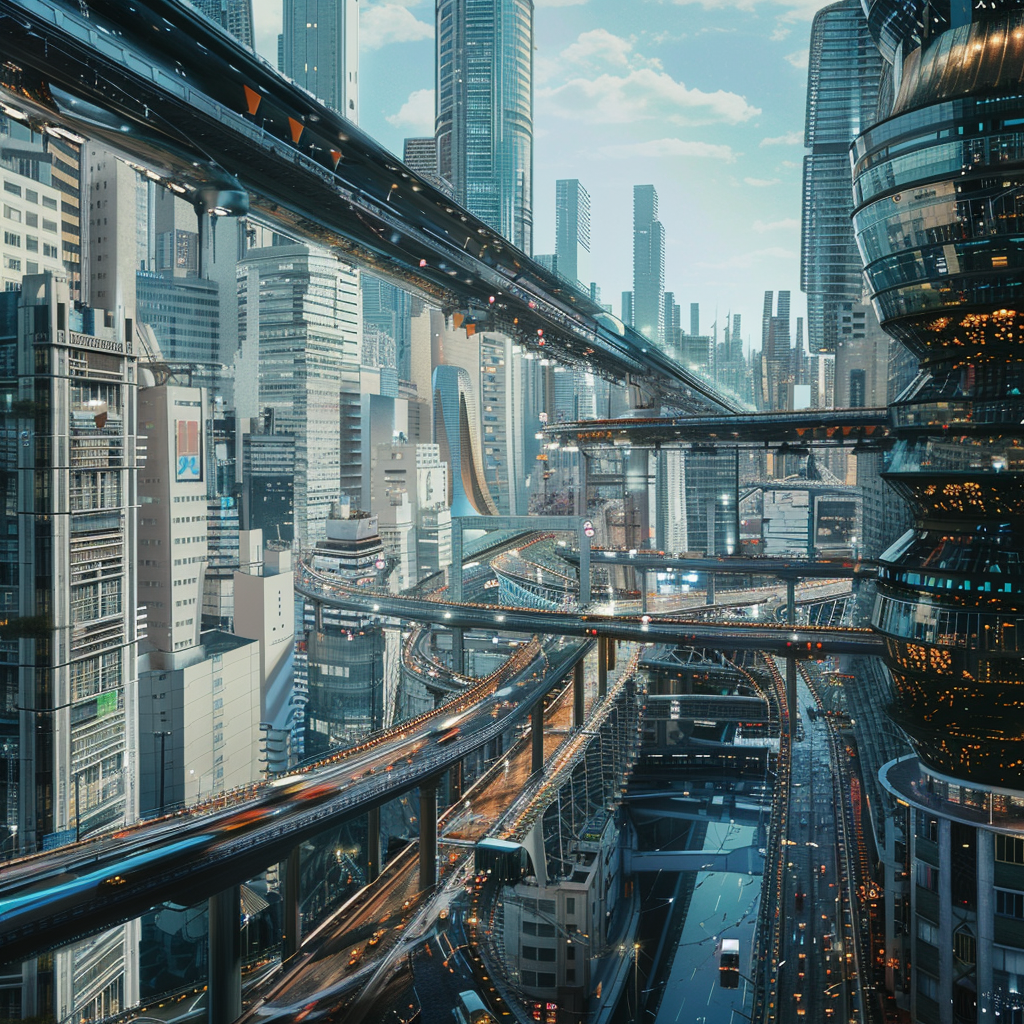
From Poster to Problem Child
From 2014 to 2022, SaaS companies were the darlings of listed tech stocks. With sticky recurring revenues and consistent revenue growth rates (the median SaaS company in the EMCLOUD index grew 30-35%/year), SaaS seemed like the safest of bets. Some people started to think of SaaS companies as the perfect financial instrument. Here was finally something that provided both the stable cash flows of a bond and the perpetual revenue growth of tech. What was not to like?
Since then, SaaS has come back down to earth. In 2024, the median revenue growth of EMCLOUD companies dipped below 20%. And while this is still growth, the trend has some folks worried. Cue median revenue multiples, which have dipped below 6x (for comparison, Nvidia is trading at nearly 30x revenue).
Have corporates stopped spending?
It’s not that corporate buyers have stopped buying technology. It’s just that the focus has shifted. And right now, all focus is on AI. As we speak, corporates are maniacally focused on “bringing AI into their enterprise operations”. Besides Nvidia, the main beneficiaries are Microsoft and OpenAI which are selling access to GPT on an API basis. And all other “non-AI” tech projects are being scrutinised and put on hold. This is leading to longer sales-cycles and lower win-rates.
But just as AI has started dominating the headlines, we are seeing a slowdown in the strength of core AI models (the LLMs that power much of generative AI – like GPT from Open AI and Gemini from Google). While they are still getting better, Emergence argues in a recent publication that performance growth has plateaued.
What – no more improvements to AI models?
To date, LLMs have mainly been trained on public data. As OpenAI, Google, and others have reached the point where their models are trained on all the publicly available data, where is the next step in model performance going to come from?
The answer lies in private data. The data residing inside enterprises, and which is going to unlock the next level of progress for AI models.
In the context of AI, private data is powerful for two reasons:
- It’s proprietary, so it can be used to differentiate models. If all models train on the same public data, they all become identical and commoditised. But private data can set them apart, making them far more valuable. Private data is the road to competitiveness and profit.
- Proprietary business data is the data that’s needed to truly automate business using AI. The knowledge of an LLM trained on public data is a bit like the knowledge of a well-studied graduate entering the corporate world for the first time. They need some time as trainees before they really understand what’s going on inside a company (and can add meaningful value). The analogy of this is “access to private data”. It’s all the stuff you can’t find in textbooks. Once we train models on private data, they will start being able to solve many more business problems. And this is not confined to writing text. It also applies to designing, operating and optimising systems in construction, manufacturing, medical research etc.
So, the truth is that, while the development of generalised LLMs might be slowing, we have barely started scratching the surface of what we can do with AI models.
Opportunity for AI-powered SaaS ahead.
And what does this mean for software and SaaS? The opportunity to develop enterprise platforms that learn from real business data is vast. Some speculate that all companies will want to do this in-house, rendering independent software vendors irrelevant. In my view, that’s essentially rehashing the argument from the open-source discussions 15-20 years ago. Some folks thought that open-source would make it so easy to make software that all companies would make their own, and that nobody would be able to make money building and selling SaaS. Since then, the SaaS industry has grown by hundreds of billions in annual revenue.
Not all of the new revenue will be in the form of vertical applications. Some of it is effectively helping enterprises make, manage or improve their own models (like our portfolio companies Octaipipe and Hirundo). But once we are through the current AI experimentation phase and as AI-powered SaaS applications start generating real ROI for users, we will see SaaS revenue growth start picking up again.